The Problem
Discovering advanced persistent threats (APT) is, by design, akin to finding a needle in a haystack.
The threat actors behind APTs combine multiple tactics, techniques, and procedures (TTP) over extended periods of time to compromise and maintain access to their targets.
The IBM Cost of Data Breach Report 2021 reported an average attacker dwell time of 212 days.
APTs evade legacy security solutions which rely on time-batched loads of data that filter for Indicators of Compromise (IoC) by executing incremental actions spread across numerous systems at rates that exceed batch analysis size and time boundaries. APT detection requires a new approach.
The Solution
Matured within DARPA’s Transparent Computing program specifically for the detection of APTs, Quine and Novelty Detector work together to efficiently uncover the aspects of advanced persistent threat detection.
Quine’s graph data model uses categorical data other systems ignore and excels at correlating individual events occurring in their billions/trillions across devices, software and services over any time period to find the behavior patterns (Indicators of Behavior or IoBs) that represent malicious activity.
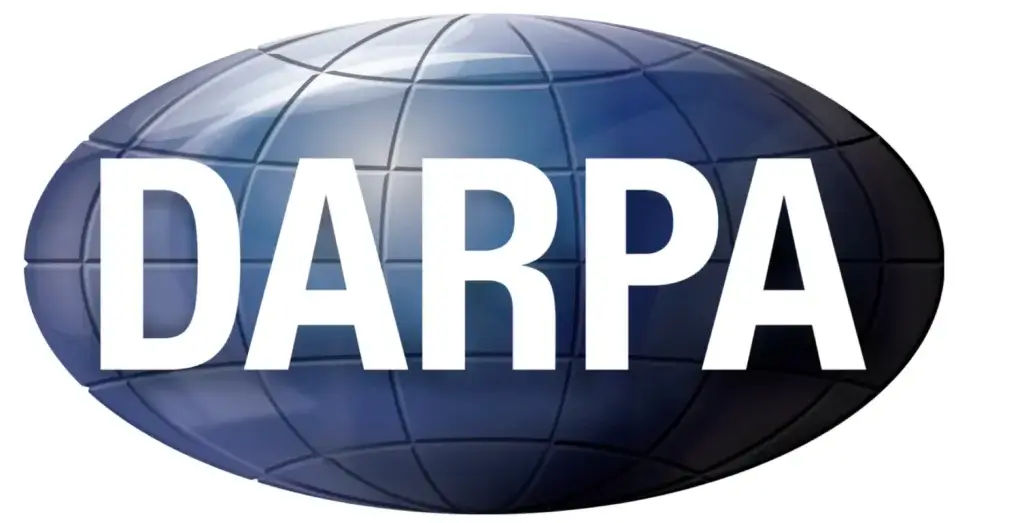
When patterns are detected, Novelty Detector can then apply its categorical anomaly detection techniques to identify when a string of related actions represents a novel/anomalous behavior, greatly reducing false positives.
Quine Enterprise provides commercial support and licensing for clustered Quine and Novelty Detector. You can add real-time behavior-based APT detection to your stack at scale and with confidence.
thatDot’s core technology underpinning Quine and Novelty Detector was developed in partnership with DARPA. Read more about thatDot’s origin and some examples of using Novelty Detector to detect data exfiltration and credential theft.
Key Value Take Away
- Quine + Novelty Detector detect both known and emerging behavioral patterns in a single workflow.
- Joins multiple data sets to enable real-time identification of attack behaviors across domains
- Identify behaviors over extended time periods using incremental streaming analysis (not batch)
- Native support for categorical data simplifies operations and provides human-readable alerts for analysts
- STIX Compliant, real-time detection of Indicators of Behavior (IoBs) and generation of STIX message events